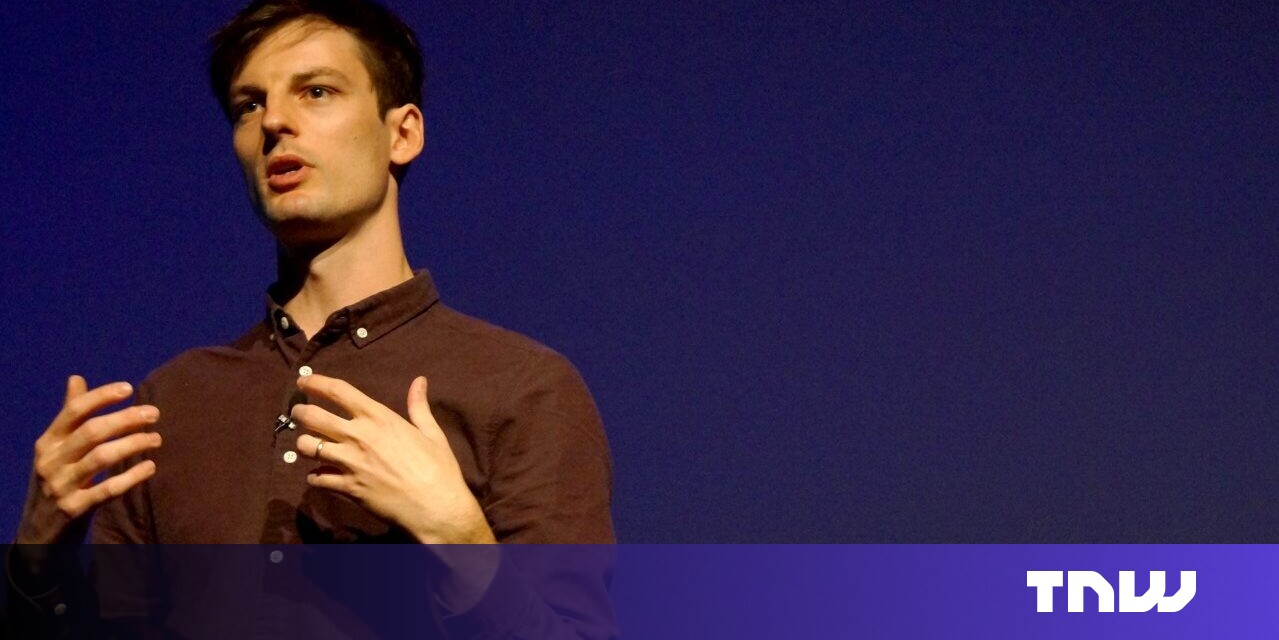
Ed Newton-Rex had reached a breaking point. As the vice president of audio at Stability AI, the 36-year-old was at the vanguard of a revolution in computational creativity. But there was growing unease about the movement’s strategy.
Stability was becoming an emerging powerhouse in generative AI. The London-based startup owns Stability Diffusion, one of the world’s most popular image generators. It also recently expanded into music generators with the September launch of Stable Audio — a tool developed by Newton-Rex himself. But these two systems were taking conflicting paths.
Stable Audio was trained on licensed music. The model was fed a dataset of over 800,000 files from the stock music library AudioSparx. Any copyrighted materials had been provided with permission.
Stable Diffusion had gone in a different direction. The system was trained on billions of images scraped from the web without the consent of creators. Many were copyrighted materials. All were taken without payment.
These images had taught the model well. Diffusion’s outputs pushed Stability to a valuation of $1bn in a $101mn funding round last year. But the system was attracting opposition from artists — including Newton-Rex.
GenAI’s ethical dilemma
A pianist and composer as well as a GenAI pioneer, Newton-Rex was at odds with the unsanctioned scraping.
“I’ve always really wanted to make sure that these tools are built with the consent of the creators behind the training data,” he tells TNW on a video call from his home in Silicon Valley.
Stability was far from the only exponent of this method. The image generators MidJourney and Dall-E apply the same approach, as do OpenAI’s ChatGPT text generator and CoPilot programmer. Visual arts, written works, music, and even code are now constantly being reworked without consent.
In response, creators and copyright holders have launched numerous lawsuits. They’re angry that their work is being taken, adapted, and monetised without permission or remuneration. They’re also worried that their livelihoods are at stake.
“It’s in the AI industry’s interest to make people think that only the big players can do this.
Artists say that generative AI is stealing their work. The companies behind the systems disagree. In a recent submission to the US Copyright Office, Stability argued that the training was “fair use” because the results are “transformative” and “socially beneficial.”
Consequently, the company asserted, there was no copyright infringement. The practice could therefore continue without permission or payments. It was a claim that had become common in GenAI, but one that Newton-Rex disputed.
“It really showed where the industry as a whole stands right now — and it’s not it’s not a place I’m happy with,” he says.
Newton-Rex considers the practice of exploitation. Last week, he resigned from Stability in protest.
The departure doesn’t mean that Newton-Rex has quit generative AI. On the contrary, he plans to continue working in the field, but following a fairer model. It’s not the impossible mission that the GenAI giants might depict. In fact, it’s already been accomplished by a range of companies.
Alternatives are available
Newton-Rex has a long history in computational creativity. After studying music at Cambridge University, he founded Jukedeck, a pioneering AI composer. The app used machine learning to compose original music on demand. In 2019, it was acquired by TikTok owner Bytedance.
Newton-Rex then had spells as a product director at Tiktok and a chief product officer at Voicey, a music collaboration app that was acquired by Snap, before joining Stability AI last year. He was tasked with leading the startup’s audio efforts.
“I wanted to build a product in music generation that showed what can be done with actual licensed data — where you agree with the rights holders,” he says.
That objective put him at odds with many industry leaders. GenAI was edging into the mainstream and companies were rushing to ship new systems as quickly as possible. Scraping content from the web was an attractive shortcut.
It was also demonstrably effective. At that time, there were still doubts that the licensed datasets were large enough for training state-of-the-art models. Questions were also raised about the quality of the data. But both those assumptions are now being disproved.
“What we call training data is really human creative output.
Stable Audio provided one source of counter-evidence. The system’s underlying model was trained on licensed music in partnership with the rights holders. The resulting outputs have earned applause. Last month, Time named Stable Audio one of the best inventions of 2023.
“For a couple of months, it was the state-of-the-art in music generation — and it was trained on music that we’d licence,” Newton-Rex says. “To me, that showed that it can be done.”
Indeed, there’s now a growing list of companies showing that it can be done. One is Adobe, which recently released a generative machine-learning model called Firefly. The system is trained on images from Creative Commons, Wikimedia, and Flickr Commons, as well as 300 million pictures and videos in Adobe Stock and the public domain.
As this data is provided with permission, it’s safe for commercial use. Adobe also stressed that creators whose work is used will qualify for payments.
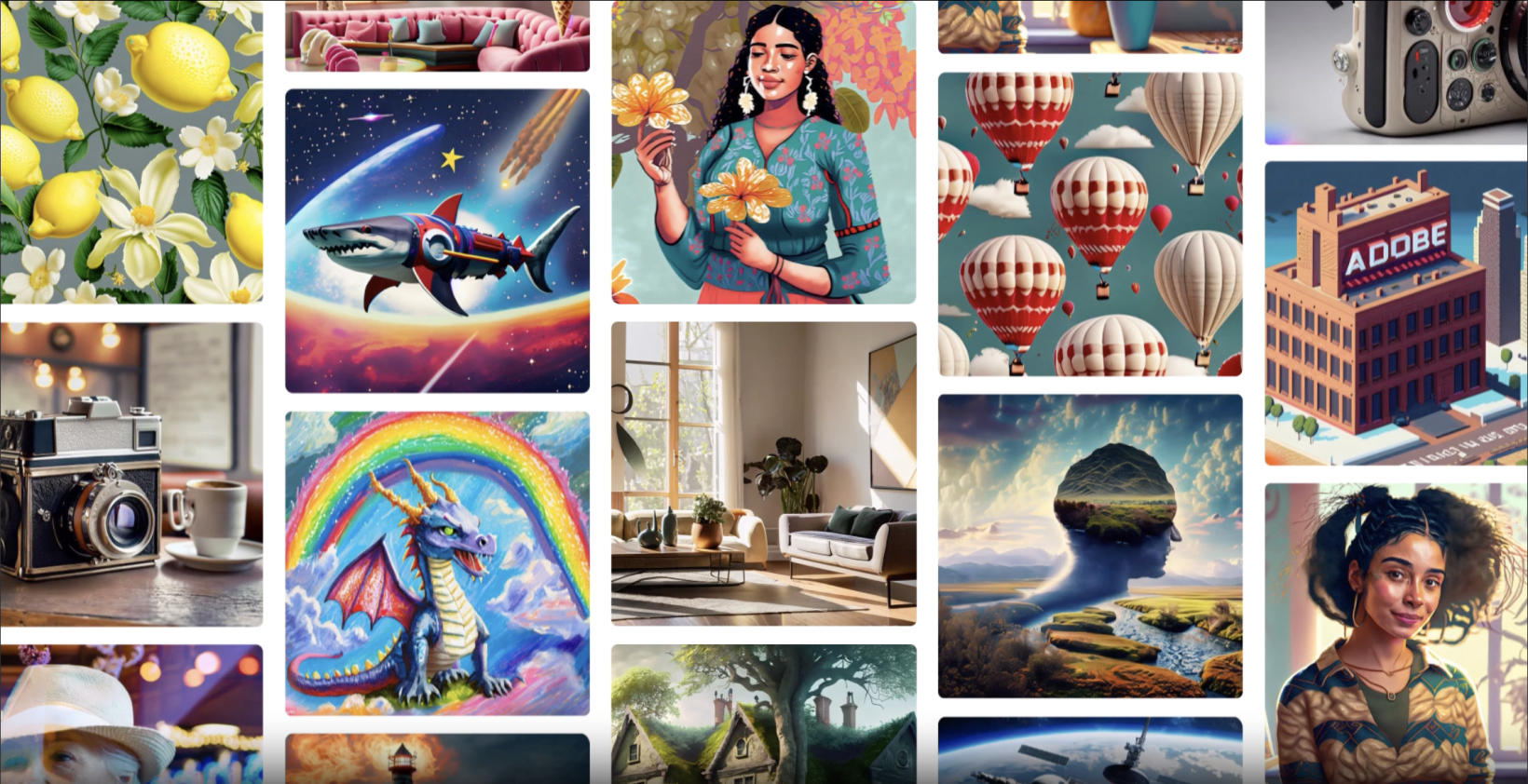
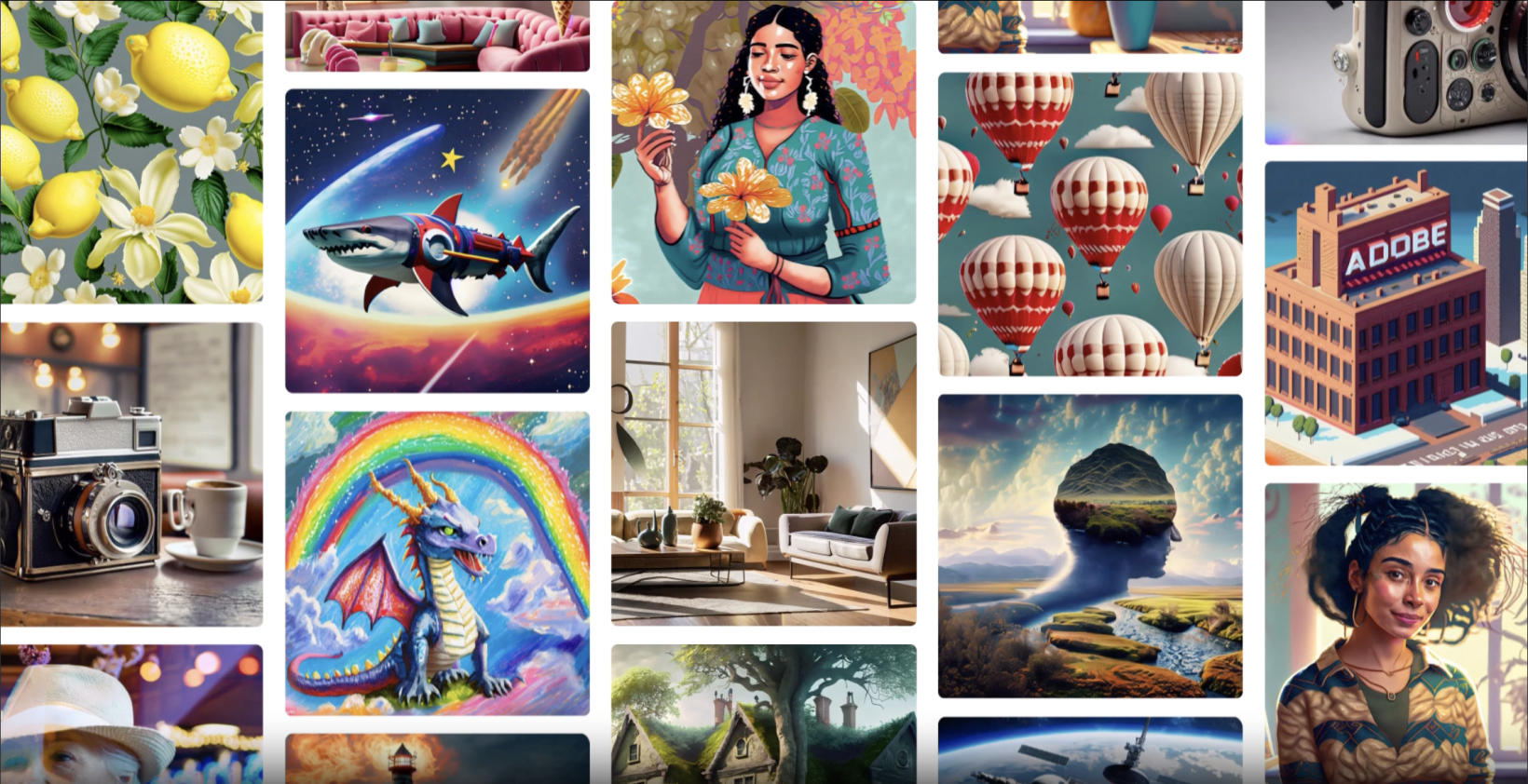
Another alternative model comes from Getty Images. In September, the company launched Generative AI by Getty Images, which is trained solely on the platform’s enormous library. Craig Peters, the firm’s CEO, said the tool addresses “commercial needs while respecting the intellectual property of creators.”
Nvidia has also developed GenAI in partnership with copyright holders. The tech giant’s Picasso service was trained on images licensed from Getty Images, Shutterstock, and Adobe. Nvidia said it plans to pay royalties.
These approaches won’t work for everyone. As mega-corps with deep content pools, the companies behind them have resources that few businesses can match. Yet startups are showing that licensing can also be done on a budget.
GenAI for the people
Bria AI has provided one example. The company has developed a new commercial open-source model for high-quality image generation. All the training is done on licenced datasets, which were created in collaboration with leading stock photo agencies and artists. A revenue-sharing model provides creators and rights-holding with compensation for their contribution
It’s a similar approach to the one Newton-Rex used at Stable Audio — but it’s not the only one.
Companies can also provide upfront payments to artists, create joint ventures that give rights holders equity in the business, or use content with a Creative Commons license, which can be freely re-used without explicit permission. GenAI firms may dismiss these efforts, but they have ulterior motives.
“It’s in the AI industry’s interest to make people think that only the big players can do this — but it’s not true,” Newton-Rex says.
“You might need to get a little inventive. You certainly have to do some negotiations and be willing to spend the time. But ultimately, what we call training data — and what is really human creative output — is a resource for tech companies. They need to work to get that in the same way they need to work to get any resource.”
If they’re willing to do that, GenAI can work in harmony with human artists. And hopefully, let all of us enjoy the creativity unleashed by them both.